Machine Learning – what it is and why your Hotel needs to use it.
Machine Learning (ML) and Artificial Intelligence (AI) have been buzzwords for a while, but what do they mean to you and your hotel operations? We all have experienced and appreciated voice recognition systems because they improve our experiences. Amazon Echo and Google Home are well-known examples of consumer goods with embedded AI capabilities. Real-time fraud detection and purchase recommendation engines are also good examples of AI and ML in action.
What may not be so clear is how ML fits into our day-to-day world of Data Analytics. This paper is part of a series, sponsored by HEDNA’s Hotel Analytics Working Group, that aims to deliver a high-level understanding to hoteliers working as analysts within hotel distribution, sales and marketing and revenue management.
The hospitality industry, especially in these challenging times, needs to embrace better ways of interpreting data to understand customers’ needs and buying behaviors. This article covers the basics of ML to better understand how it can be used to achieve positive business outcomes.
Why ML?
Business competitiveness today relies on taking timely actions in response to changing circumstances. IT systems have historically automated redundant tasks. Recently, new analytic systems are being built that automatically factor in changing circumstances to understand the impact, learn from them, and make necessary adjustments.
The expectations are that systems will supply increasingly higher quality analysis faster and at greater scale. Many of today’s systems are already fulfilling this need to the point of automating reactions and learning how to improve these reactions over time.
According to Scott Boettcher, VP of Data Science at NTT DATA, “In order to adapt and adjust to changes we see enormous potential from two ML dynamics:
- the aggregation of narrow intelligence systems assembled into a super intelligence. Each of the different components involved in this challenge taps into deep learning capabilities and, when combined, the resulting system can rival or exceed human performance.
- the human should not completely leave the equation. Instead, we must think in terms of mutual augmentation.”
In terms of mutual augmentation, a Human-in-the-Loop (HITL) is vital, crucial in training the algorithms to better interpret the patterns, and increase the chance of first catching, then adjusting to the emergent patterns.
Where ML fits into Analytics
To understand how ML fits within Analytics, it is helpful to think of analytics as a series of progressive steps. Figure 1 depicts that progression using ladder steps, maturing from level 1, manual Descriptive Analytics to Level 5, automated Cognitive Analytics.
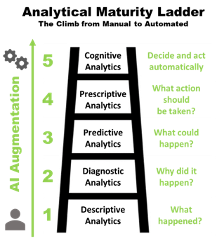
Figure 1: Analytical Maturity
ML belongs to the category at the top rung of the ladder “Cognitive Analytics”. Much of the tremendous growth in Data Analytics is attributable to the growth in Cognitive technologies. AI and ML are chief among the growth technologies with a compound annual growth rate over 42%.[1]
Analytics is increasingly reliant on Big Data and ML is no exception. ML assists cognitive technologies to learn intelligently. ML algorithms need copious amounts of data for the algorithms to learn the data patterns and behaviors. Extract Transform Load (ETL) processes (and variations such as ELTL) will feed analytical capabilities for large data sets to predict patterns, trends, and associations. The output of this predictive analysis can be a prescriptive engine (ladder rung #4). The engine then “trains” the AI to make intelligent decisions that can match or exceed the quality of the human decisions. The relevance of a best practice framework as described in Figure 2, is more important than ever to advance up the Analytical Maturity Ladder using ML.
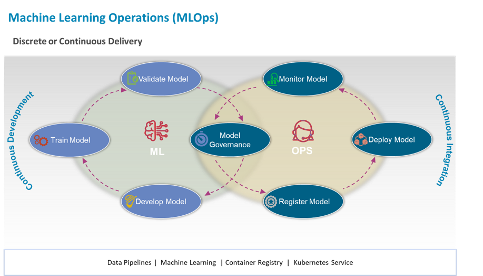
Figure 2: NTT DATA Intelligent Data Services – Machine Learning
Types of ML
The number of technologies that are “cognitive” are growing. Today’s CIOs will typically encounter applications that are based on machine learning and/or deep learning neural networks. Both types of learning help to speed up processes and mimic the functioning of the human brain to increase automation and decision-making capability.
- ML is the ability of statistical models to improve performance over time without the need to follow explicitly programmed instructions.
- Deep Learning is a complex form of ML involving neural networks, with layers of abstract variables. Deep learning models are excellent for image and speech recognition.
These two forms of “learning” are often combined with other forms of AI to create improved and smart systems as shown in Figure 3, “Types of Learning as the Base of AI”.
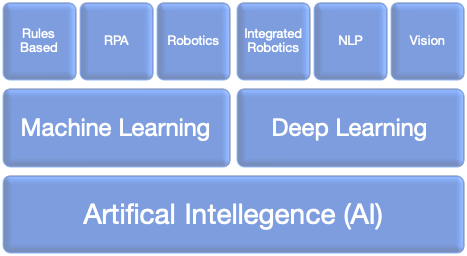
Figure 3: Types and uses of Learning in AI
- Rules-based systems is the ability to use databases of knowledge and rules to automate the process of making deductions and inferences about information.
- Robotic process automation (RPA) is software that automates repetitive, rules-based processes usually performed by people sitting in front of computers. By interacting with applications just as humans would, “bots” can open email attachments, complete e-forms, record, and re-key data, and perform other tasks that mimic human action.
- Physical robots can perform different physical tasks often in collaboration with human workers. The broader field of robotics is embracing cognitive technologies to create robots that can work alongside, interact with, assist, or entertain people.
- Natural language processing/generation (NLP/G) is the ability to extract or generate meaning and intent from text in a readable, natural, and understandable form.
- Speech recognition is the ability to recognize and transcribe human speech.
- Computer vision is the ability to extract meaning and intent out of visual elements. Examples include characters (in the case of document digitization), faces, objects, and activities.
Leveraging ML
Within the field of ML, there are two main types: structured & supervised, and unstructured & unsupervised:
- Structured learning, as the name suggests, is when the machine learns using data which is already labelled and tagged and where the outcome is determined by pre-set values. Structured learning is already a component of many Revenue Management for distribution
- Unstructured learning by contrast is the training of the machine without guidance. Using information that is neither classified nor labeled, the task of the machine is to group unsorted information according to similarities, patterns, and differences without prior training of data.
Unstructured learning can discover hidden patterns and structures. These discoveries can lead to actionable insights. These algorithms seek to find the underlying structure or pattern of a data set without knowing the answer in advance.
Driven by the COVID-19 pandemic crisis, hoteliers are increasingly aware of AI solutions that can help them through recovery and beyond. Property operations are turning to automation as a way of enhancing their property monitoring and security. Computer vision analyzes visual data in real-time generating triggers for HITL interaction when required. Other industries like manufacturing and retail are moving towards “Back to Work” solutions with enforcement of mask detection using computer vision and facial recognition.
These systems automate the use of external data or learnings in their decision-making process. Weather, macro-economic, and Covid-19 related data allow for better financial planning as well as in the design of strategic supply chains.
Yield management is a classic example, where AI can help hoteliers achieve improved yield through realizing:
- Optimized profit and revenue calculations
- Utilization control and management of facilities. (conference halls, restaurant covers, public spaces, casino gaming areas)
- Improved distribution channel optimization
Other ways that ML can be leveraged include answering questions such as the following:
- How is profit affected by changes in rates, and what rate results in the highest profit?
- During seasonal peak demands, can profitability be maximized while optimizing staffing and resource levels, from both a quality of service and cost perspective?
- During seasonal peaks, can demand and profit be forecasted while factoring in competitor prices, promotions, and the risk of adverse events?
- Can a better understanding of customers be obtained by using AI & ML to automatically classify and segment customers and prospects in deeper and yet unseen ways? Would doing so allow personalized offers and services, leading to increased customer happiness, and ultimately great profits?
- What is the impact of social media and can it and other external sources be used in real-time to realize improvements?
By automating the AI & ML that answers these questions and solves these problems will enable decision makers to take advantage of these improvements in real time.
Goals for ML and Cognitive Analytics: Smarter Insights, Stronger Outcomes, and a Self-driving Business
Hoteliers ultimately want to increase the value of their hospitality services for their customers. They can achieve this through making the systems they depend on for acquiring and servicing those customers “smarter”. AI can improve decision-making by sifting through large volumes of data to find timely answers. This leads to better business outcomes. For example, instead of manually drilling into spreadsheets or dashboards, Revenue Managers or Distribution Executives will be able to ask questions about their company’s financial performance using a cognitive tool that combines voice recognition capabilities with flash reporting.
Companies are also using cognitive technology to augment human judgment by helping their staff make better decisions. Better decisions are key drivers for hoteliers increasingly adopting AI. Scott Boettcher observes that: “digital decisioning begins with Business Analysts who identify the critical decisions humans make within a business process, but which alternatively, can be made more effective through AI.” To accomplish this, Boucher recommends the process shown in Figure 4 to enable a “Self-Driving Business”.
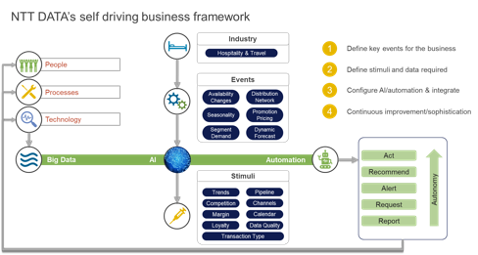
Figure 4: NTT DATA Self Driving Business
The vision of using AI and ML, and of ultimately attaining a Self-Driving Business, is to build a business that minimizes costs wherever possible, is reactive as possible, and drives optimization in all its forms. These could be maximizing profits, promoting the best packages, services the customers in the best way, etc. Even with all the promise, business experts are still required to chart the journey by training the machine and ultimately achieving an ongoing process of augmented machine learning.
[1] Forbes “Roundup of Machine Learning Forecasts And Market Estimates, 2020”
Written by Criss Chrestman, Advisory Services, Technology & Innovation Commercial Industries , NTT Data Services
Edited by Sonja Woodman, Independent Travel Tech Writer